Taste testers aside, there's a new contender in the world of taste assessment – and it's made of circuits and sensors.
And eventually, scientists say the technology could be used to find contaminants in foods that could make consumers sick or detect when something is no longer safe to eat.
Researchers at Penn State in Pennsylvania have developed an electronic tongue that identifies differences in similar liquids, such as milk with varying water content, and products such as types of soda and coffee mixes. It can even distinguish between Pepsi and Coca-Cola with a high degree of accuracy.
The research, published Wednesday in Nature, found that the AI tongue is capable of detecting, classifying and evaluating the quality and freshness of different substances. This means that if the milk is contaminated, the tongue can detect it.
“We're trying to make an artificial tongue, but the process of how we taste different foods involves more than just the tongue,” said corresponding author Saptarshi Das, professor of engineering sciences and mechanics at Penn State.
“We have our own tongue, made up of taste receptors that interact with food species and send their information to the taste cortex – a biological neural network.”
The electronic tongue comprises a graphene-based ion-sensitive field-effect transistor, or a conductive device that can detect chemical ions, linked to an artificial neural network trained on multiple data sets. It is located in the top right corner of the device.
Saptarshi Das Laboratory/Penn State
The taste cortex, located in the brain, perceives and interprets flavors beyond the basic categories of sweet, sour, bitter, salty and savory detected by taste receptors, the researchers explained. As the brain becomes more familiar with these flavors, it becomes better at detecting the subtle nuances between different flavors.
The researchers aimed to replicate the function of the taste cortex by developing a machine learning algorithm designed to mimic it.
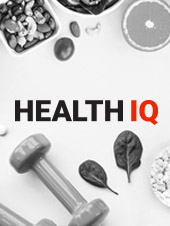
Receive weekly health news
Receive the latest medical news and health information every Sunday.
The AI's tongue is composed of graphene and chemical sensors. Chemical sensors detect and measure the chemical composition of liquids (such as carbohydrates, proteins, lipids, acids and vitamins) and convert the information into electrical signals, the researchers said.
The neural network was trained on different datasets and given 20 specific tasks to learn. These tasks dealt with how liquids affect the electrical properties of sensors.
The AI tongue accurately detected samples including watered down milk, different types of soft drinks (Diet Coke, Pepsi, Coke Zero Sugar), coffee blends (espresso, breakfast, Italian) and various fruit juices at various levels of freshness. The researchers reported that it could accurately decipher the contents in about a minute, with over 80% accuracy.

For fruit juice, the AI language achieved accuracy values of approximately 98 percent for identifying the type of fruit and 99 percent for determining its age.
“After achieving reasonable accuracy with human-selected parameters, we decided to let the neural network set its own values of merit by providing it with the raw sensor data,” said co-author Andrew Pannone, a doctoral student in engineering sciences at Penn. . State.
“We found that the neural network achieved near-optimal inference accuracy of more than 95 percent when using machine-derived merit numbers rather than those provided by humans,” he said in the media release.
By using this AI tongue to accurately detect subtle differences in liquids, potentially beyond human perception, sensors could be applied to identify harmful contaminants in food, such as PFAS (a toxic chemical), and to monitor food freshness, how to track juice quality over time. several days, the researchers argued.
“While there are corrective actions for food adulteration and contamination incidents, monitoring food freshness is more challenging due to the complex and variable chemical compositions present in food. Spoiled food is dangerous to consume and has a diminished nutritional value,” the study stated.
The authors emphasize that the timely detection of harmful contaminants in food production and distribution remains a constant challenge. For example, dangerous PFAS are widely used in industrial processes and are present in consumer products, with the potential to accumulate in the environment, including drinking water.
PFAS, known as “forever chemicals,” are a group of thousands of long-lasting, man-made chemicals used in textiles, cosmetics, furniture, paints, firefighting foams, food packaging and other products. commonly used consumer products.
Exposure to certain PFAS is associated with reproductive, developmental, endocrine, hepatic, renal and immunological effects, according to Health Canada.
However, AI language shows promise in accurately detecting PFAS in water, offering a potential solution to this problem.

“We believe that miniaturized graphene-based technology, enhanced by a suite of machine learning methodologies, can serve as a cost-effective platform for a wide range of chemical sensors.
applications in the food supply chain and beyond,” the authors state.
The researchers note that the AI language's capabilities are limited only by the data it is trained on. Although this study focused on food evaluation, its potential applications could also extend to areas such as medical diagnosis.
“These results highlight that machine learning-aided graphene ISFETs (ion-sensitive field-effect transistors) can be applied to address a broad spectrum of challenges in the food industry,” the researchers said.
— With files from Saba Aziz of Global News